Gravity R&D Inc develops online recommender systems. The Netflix Prize contest gave them the first reference, then they received research funding, among others the predecessor of the NRDI Fund. Their customers are 50% foreigners and 50% Hungarians, and no one can beat their algorithms on the market. They pretty much know what users want even if they visit the given website for the first time. They expect their revenues doubled in 2017.
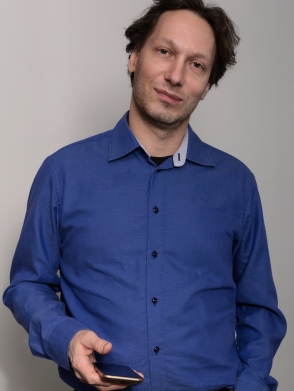
Domonkos Tikk CEO at Gravity R&D
Recommender systems are everywhere now, taking various forms: online stores, music and movie streaming service providers and social media all apply some kind of algorithms to recommend personalized and relevant products and services to the users based on different information. The potential of such systems was recognised by a team formed at the Budapest University of Technology and Economics. In 2006 they entered the 1 million dollar grand prize competition organised by Netflix, the video-on-demand provider already popular in the US but little known in Hungary at the time. The task was to develop the company’s recommender system and the team was tied for first place which earned them high prestige.
“Three of the four members of the Netflix Prize team are still active in the company which now has more than 40 employees,” says Domokos Tikk in the Budapest office about the early days of the company founded after the competition. Since ever, the company is committed to do research, they were for instance key member of CrowdRec, an international project aimed at developing recommender systems, for which they won substantial funding from the Seventh Framework Programme for Research and Technological Development (FP7) of the European Union, together with several academic and industry partners. More than EUR 300,000 was awarded in EU funding for the project which started in 2013 and ended in 2016. “We spent a large part of the money on financing research and researchers, and the necessary hardware as we had to pay high salaries to retain professionals and researchers with extremely valuable knowledge.”
The highly research intensive project was aimed at developing a recommender system which can adapt to the intent of users’ visits in real time. In practice this means that the system recommends products based on the user’s real-time behaviour (primarily the sequence of clicks) and other available information (device, browser, location). The novelty was that the real-time click sequence is integrated into the algorithm, as former recommender systems relied solely on historical data, that is, they tried to predict users’ needs based on their past behaviour.
Gravity’s deep learning based algorithms raise this to a new level by recommending relevant alternatives during a given session. “This is particularly useful when facing the so-called cold start problem when either the product or the user is new, so no historical data is available.” The first half of the project focused on the necessary basic research and resulted in the creation of the deep learning algorithm specialized for recommendation system, called GRU4Rec .
The second half was dedicated to the testing, measurement and fine-tuning of the algorithm on various reference data sets, that is, they examined how the system will perform in real-life situations. By the way, the technology is highly compute-intensive: the algorithm uses the abilities of the graphics processing unit (GPU) of the computer to create the recommendations. “This requires a high number of vector and matrix operations for which, due to their architecture, GPUs are more suitable than CPUs,” said Balázs Hidasi, head of research at Gravity.
Autonomous software
The company is working on adapting its business model in a win-win way for such customers that demand high computational capacity: this capacity is provided by Gravity through external cloud-based resources as it makes a huge difference what capacity the customer needs and how much it costs.
Besides doing research, Gravity has also been present in the market with its personalization service called Yusp that is already used by several smaller and bigger companies in 25 countries worldwide. They always perform outstandingly in quality tests and tenders. Domonkos Tikk proudly notes that they have never been beaten by competitors our clients’ in-house solutions in any A/B tests (when different solutions are compared). When a new customer arrives, they first train the system by uploading all the information and data provided by the customer. Next, the software can operate fully autonomously but if necessary it can be fine-tuned for marketing demands like promotions, clearance sale and Black Friday events. In such cases, the personalization system can be adjusted to achieve the special targets effectively. The settings can be accessed through a control panel, and it is up to the customer to decide whether they do it for themselves or commission Gravity’s experts to make the modifications.
The regular business model is simple: in most cases the price is proportional to the traffic which is based on the number of recommendations served by the system, but sometimes pricing may also depend on additional KPIs, so the customers are charged based on the extra revenue generated by the personalization system.
Although the company’s customers are 50% Hungarians and 50% foreigners, their revenue mostly come from the latter group. Domonkos Tikk says foreign companies gave around 95% of their revenues and also reveals that they closed a very successful year in 2017: they doubled their previous year’s revenues (HUF 632 million, EUR 2 million)) and intend to follow this growth trajectory in the years to come. They do not plan to push research into the background either as this is the only way to remain one step ahead in the game in a dynamically developing market.
Gravity successfully runs for Hungarian RDI funding and also for received funding for their development under several schemes of the National Research, Development and Innovation Fund and its legal predecessors:
5LET_08-5LET_08_2-2009-0029
The development of a recommender system algorithm providing recommendations for cultural goods and cultural events. In the case of cultural goods, the aim is to understand users’ browsing habits by mining data from weblogs and then to use product and user metadata in recommender algorithms, and finally to develop a learning algorithm for optimising parameters and algorithm components. In the case of events, the recommender algorithm relies on data mined from the metadata and description of the event and from word clouds.
EUREKA_HU_12-1-2012-0008
The displaying of cultural and leisure activities in a user-friendly way and providing personalised recommendations. Recommendation of collaborative activities taking into consideration the preferences of multiple users (the MAGICIAN project).
Awarded funding: HUF 32,895,386
Implementation:
- classification, structural description and labelling of cultural events;
- creation of databases for personal user profiles;
- development of a family of algorithms;
- MAGICIAN application.
EU_KP_12-1-2013-0032
CrowdRec: customising digital media content through a crowdsourcing based recommender system for social networks. The algorithms to be developed are aimed at creating a symbiosis between users and content, as well as building relationships which satisfy users’ demand for content but also recommend such content to users expected to enter into interactions which facilitate the better digital understanding and description of media content.
Received funding: HUF 3,588,260
Result of the project: Preparation and submission of the project proposal “CrowdRec – Crowd-powered recommendation for continuous digital media access and exchange in social networks” for the FP7-ICT-2013-10 call.